
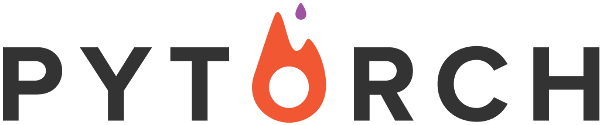
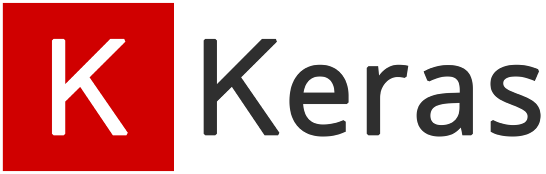


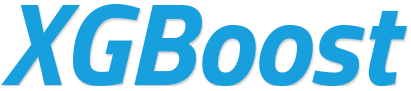
Guild AI brings systematic control to machine learning to help you build better models faster. It's freely available under the Apache 2.0 open source license.
Track Experiments
Guild automatically captures every detail of your training runs as unique experiments.
Compare and Analyze Runs
Use results to deepen your understanding and incrementally improve your models.
Tune Hyperparameters
Find optimal hyperparameters without setting up complicated trials.
Automate Pipelines
Accelerate model development, eliminate costly errors, and measure results.
Train and Backup Remotely
Train on GPU accelerated systems running on any cloud or on-prem environment.
Publish and Share Results
Share your results with colleagues through HTML, Markdown or LaTex based reports.
Command Line Interface
Use your current console based work flow with Guild's POSIX compliant interface.
Jupyter Notebook Integration
Use Notebooks or interactive shells with Guild's Python interface.
Control and Measure
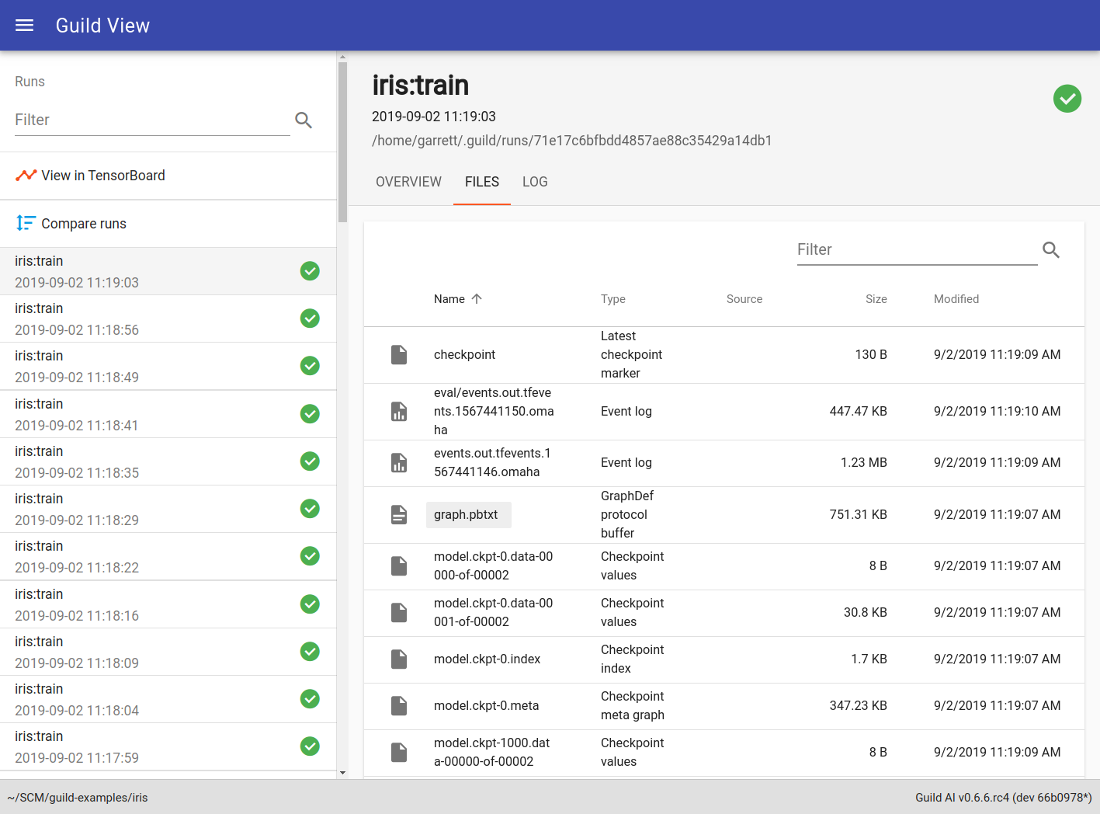
Record each operation, including source code, hyperparameters, results, and generated artifacts. Build with confidence having full governance and auditability.
Go Beyond Notebooks

Run your Notebook code with Guild to record summaries over the entire life cycle of your models. Know what changed three hours ago, or three years!
Tune Hyperparameters
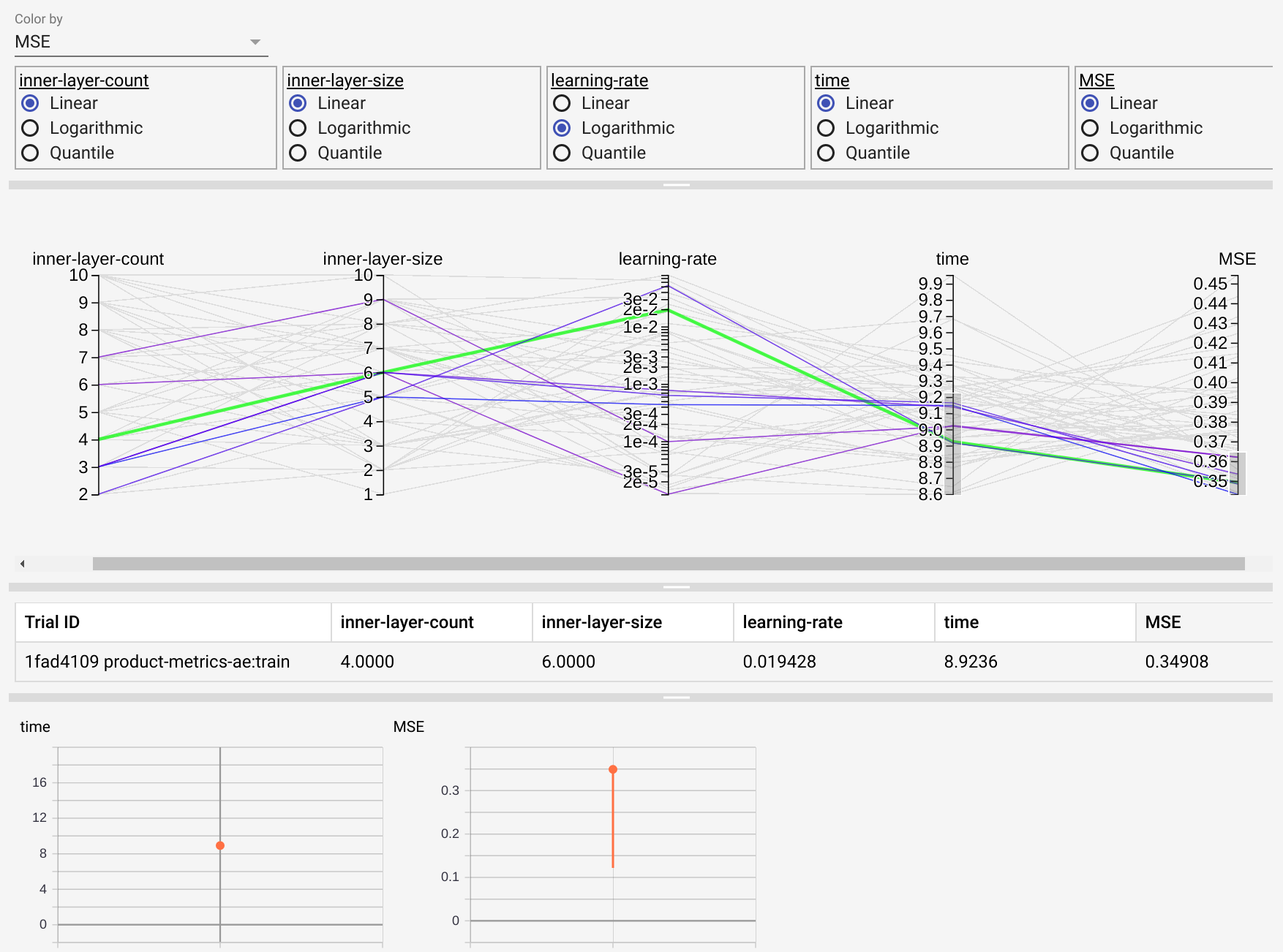
Apply state-of-the-art tuning algorithms to your models with simple commands. Easily optimize with upstream changes to data or model architecture.
Compare and Diff

Identify fine grained differences across runs to resolve complex issues and give data scientists the feedback needed to make informed decisions.
Automate Tasks
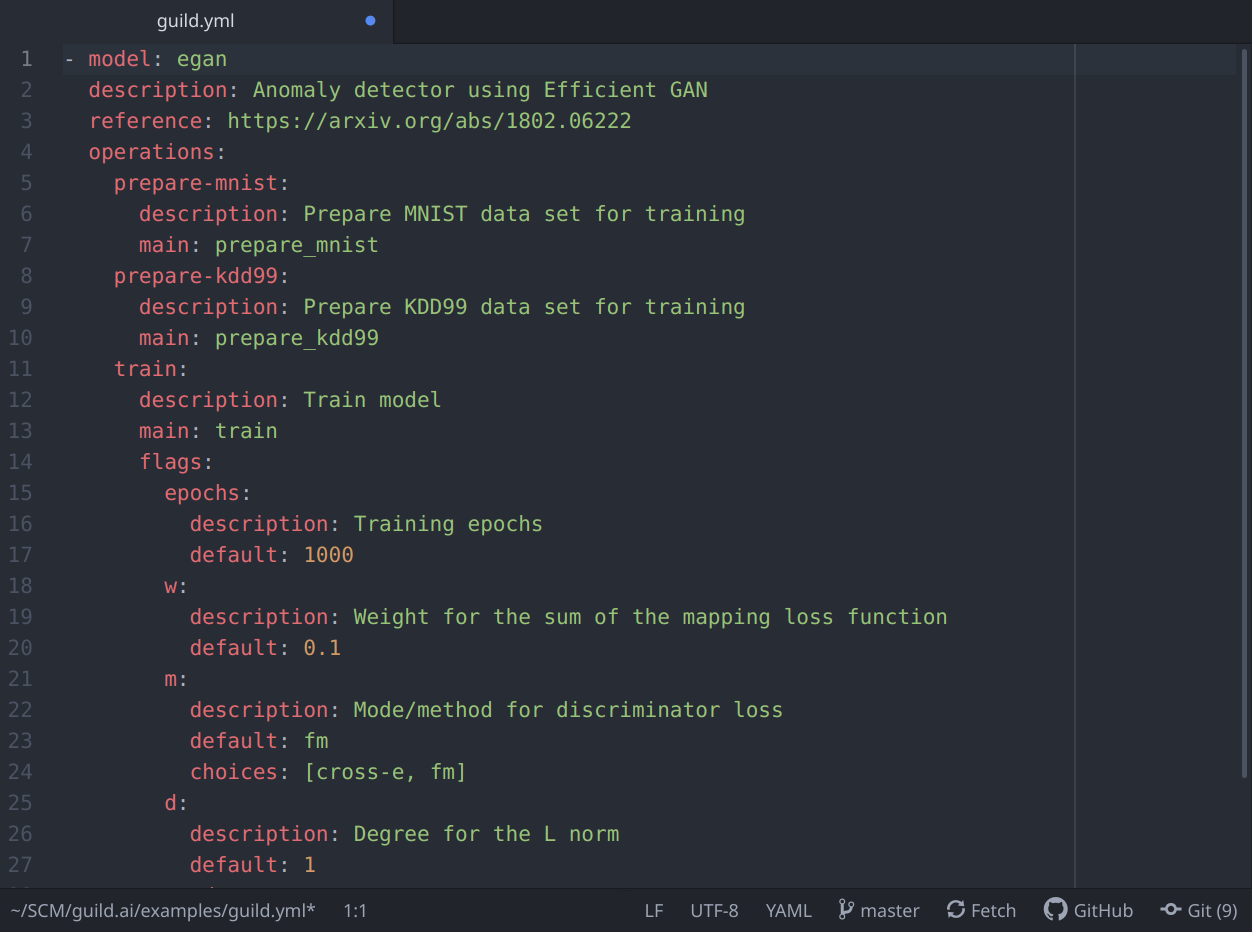
Guild supports a simple configuration scheme that lets you define model operations that perform complex tasks with a single command.
Best Practices
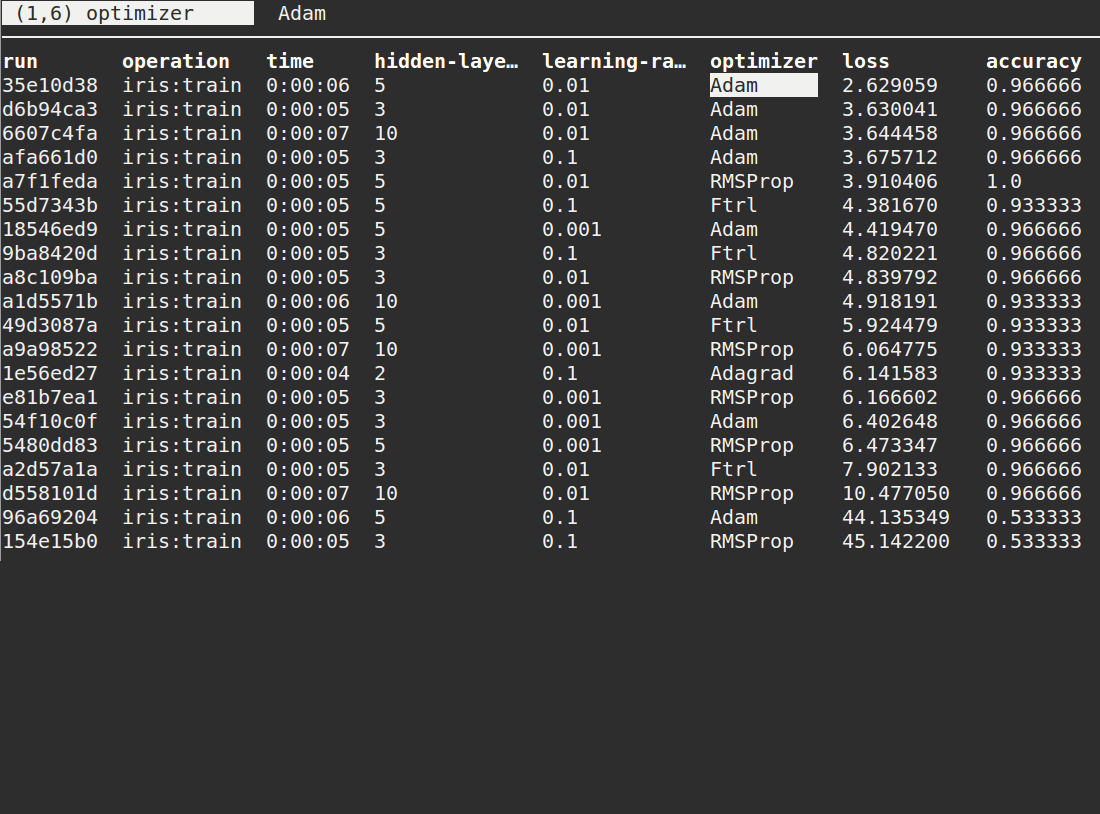
Integrate Guild features into any environment without reengineering effort. Commands are POSIX compliant and run without external dependencies.